Secure Modeling and Intelligent Learning in Engineering Systems Lab
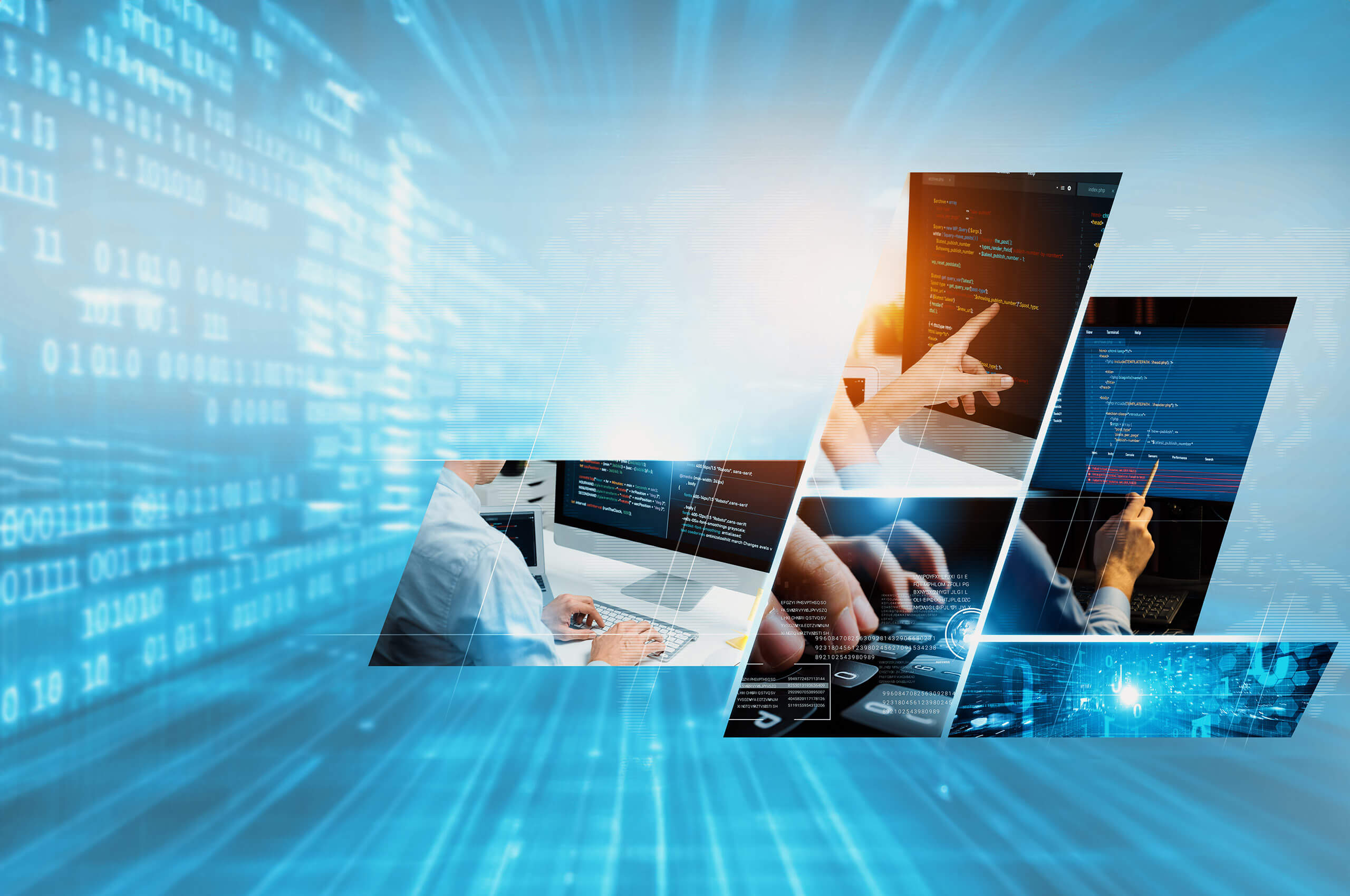
AI Tools for Cybersecurity & Neurodegenerative Diseases
Director and PI: Khalid Malik, Director of Cybersecurity Programs, Professor, Computing Division, CIT
The Secure Modeling and Intelligent Learning in Engineering Systems (SMILES) Lab is a forward-thinking interdisciplinary group of faculty and student researchers who are embracing outside-the-box thinking to develop cutting-edge AI-based solutions to some of the most pressing problems of our time. The translational research put forth by the SMILES team has an impact that extends beyond our community with marketable solutions in cybersecurity and healthcare that will benefit us all.
The bold vision of the SMILES lab has identified pressing needs and put unwavering focus on building and improving AI tools to solve them. Malik and his team have published many journal and conference articles, and they are continually building on that foundation. Through rich relationships with industry and medical experts, the team has been able to meet very specific needs with relevant solutions.
In addition to the external impact of SMILES research, the students working with SMILES projects are gaining a wealth of unique experiences. They are exposed to the latest in AI and cybersecurity tools, while constantly being supported to practice nimble, critical thinking that unlocks life-changing growth. With thoughtful mentorship from Malik, the students are empowered to practice persistence toward important tangible goals. These skills and the relationships they form will be lifelong and prepare them for a world that has much need for creative individuals who know how to bridge the gap between research and practice.
On This Page
- Deepfake Detector
- NeuroAssist
- AI-based Web Filtering
- Automated Knowledge Graph Curation
- Automotive Cybersecurity Education
Development of an Explainable and Robust Detector of Forged Multimedia and Cyber Threats using Artificial intelligence
Funded by the National Science Foundation and Michigan Translational Research and Commercialization
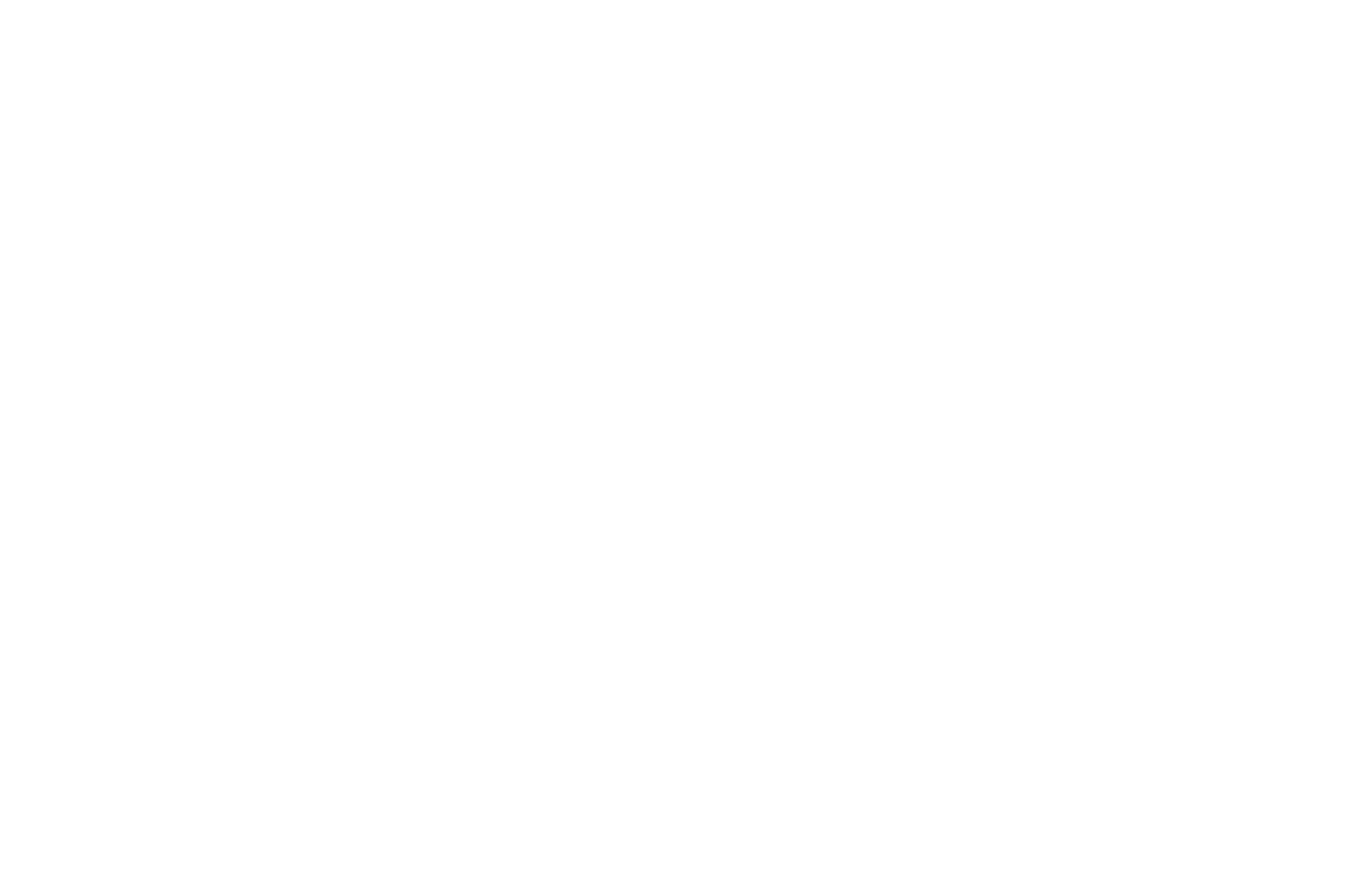
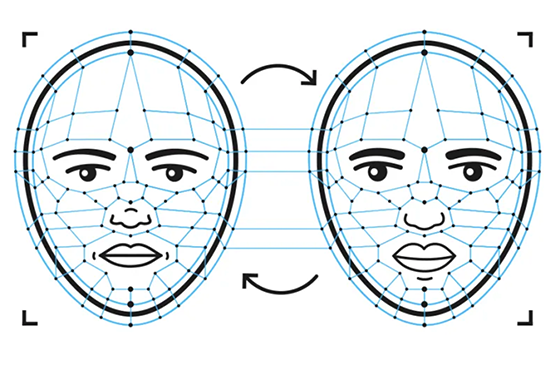

Deep Forgery Detector (DFD)
Reveal Truth. Find Justice
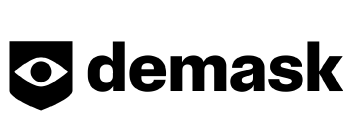
Secure Modeling and Intelligent Learning in Engineering Systems Lab
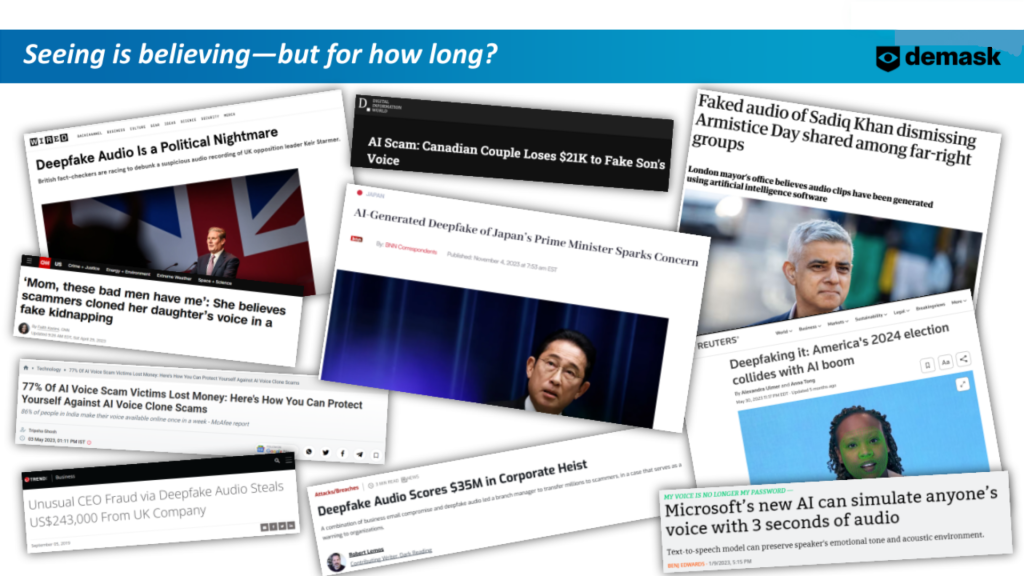
Disinformation is a growing concern for society and is fueled by a new weapon: deepfaked multimedia. We have been told all of our lives to believe what we see with our own eyes, and for the first time, we can no longer trust them. AI generated Deepfakes have left the realm of science fiction, and are an unsettling reality that demands our immediate attention.
A Deepfake is essentially a piece of media that has been either manipulated by or entirely generated by AI to appear as though it’s an original artifact. With recent developments in Generative AI tools, the capabilities have grown to the point where humans cannot detect a difference anymore without assistance.
Fake multimedia is a growing threat on the global stage. Misinformation is not a new tactic, but the tools today are far more advanced. A well-made AI video of a political or industry leader can spread false narratives about public or corporate policy and have a devastating public impact. Imagine a viral video in which some foreign head of state threatened an impending attack on the U.S. – but that video is indistinguishable from a real one.
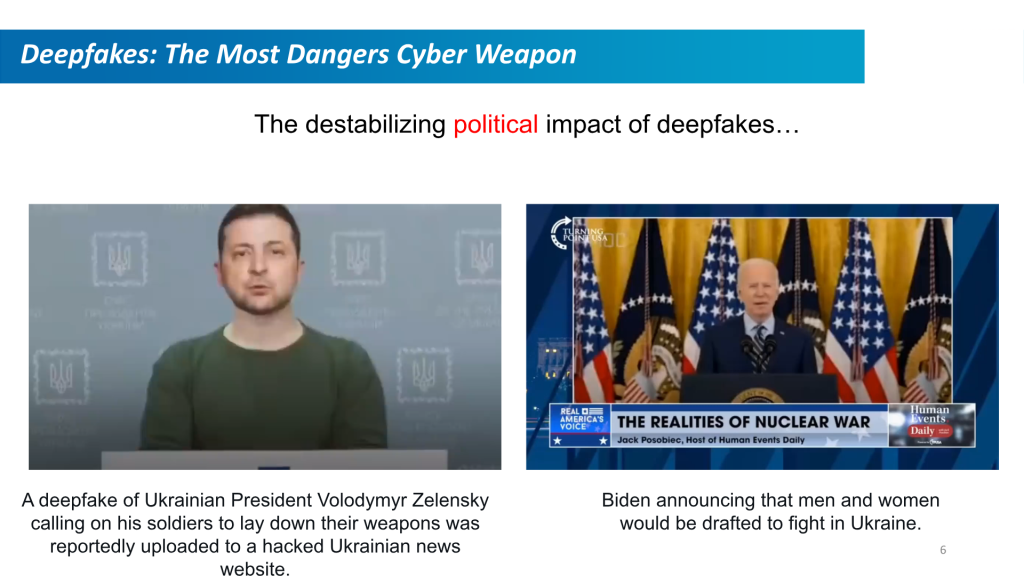
Using deepfaked audio and video in scams is increasingly possible. On Feb 4, 2024, a finance worker at a multinational firm was tricked with a Deepfake ‘chief financial officer’ video call and paid out $25 million to a scammer.
If large financial institutions can fall prey to these things, consider the vulnerability of an average citizen. According to a 2022 survey of 16,000 people across eight countries, 71% of people said that they don’t even know what a deepfake is.
As we are discussing the threat of deepfakes to global security, democratic institutions, and scams on an international level, it’s important to note that verified audio and video artifacts are now the norm as evidence in our judicial system. Deepfakes pose a significant threat to the integrity of that process.
Meeting court evidentiary standards is a challenging task, especially in the absence of underlying metadata, like digital watermarks, or if the media is post-processed with anti-forensic intent. In early February 2024, social media platforms like Meta announced that they will require AI-generated content to be labeled as such, but that falls under the category of ‘locks only keep out the honest.’ Those intent on using these advanced tools for deception will not be putting labels on them.
As the ability to create convincing fake videos has significantly increased, our need to authenticate legitimate digital media artifacts has grown as well. Beyond that, the tools needed to authenticate these media artifacts need to deliver assessments in an accessible way. Our judicial system, for example, is designed around a ‘jury of peers’ who won’t have deep knowledge of AI and cybersecurity systems.
To meet this essential demand, we have developed a Deep Forgery Detector. This research has been ongoing for over 6 years, backed by nearly $1M in grants from agencies like the National Science Foundation and MTRAC. This funding has enabled us to develop the DFD MVP with the appropriate tools and knowledge and we are working to further develop them into a product that will be usable by companies and individuals without a major background in cybersecurity.
Student researchers associated with this project will gain the opportunity to learn how to use deep learning, Neurosymbolic AI, and Multimodal AI to develop tools to authenticate digital multimedia. The students will also learn how to protect detectors from anti-forensic attacks and gain experience in designing AI-based detectors to be transparent and explainable with accessible outputs. They will get the opportunity to work in interdisciplinary teams and solve problems beyond what they would encounter in a classroom setting.
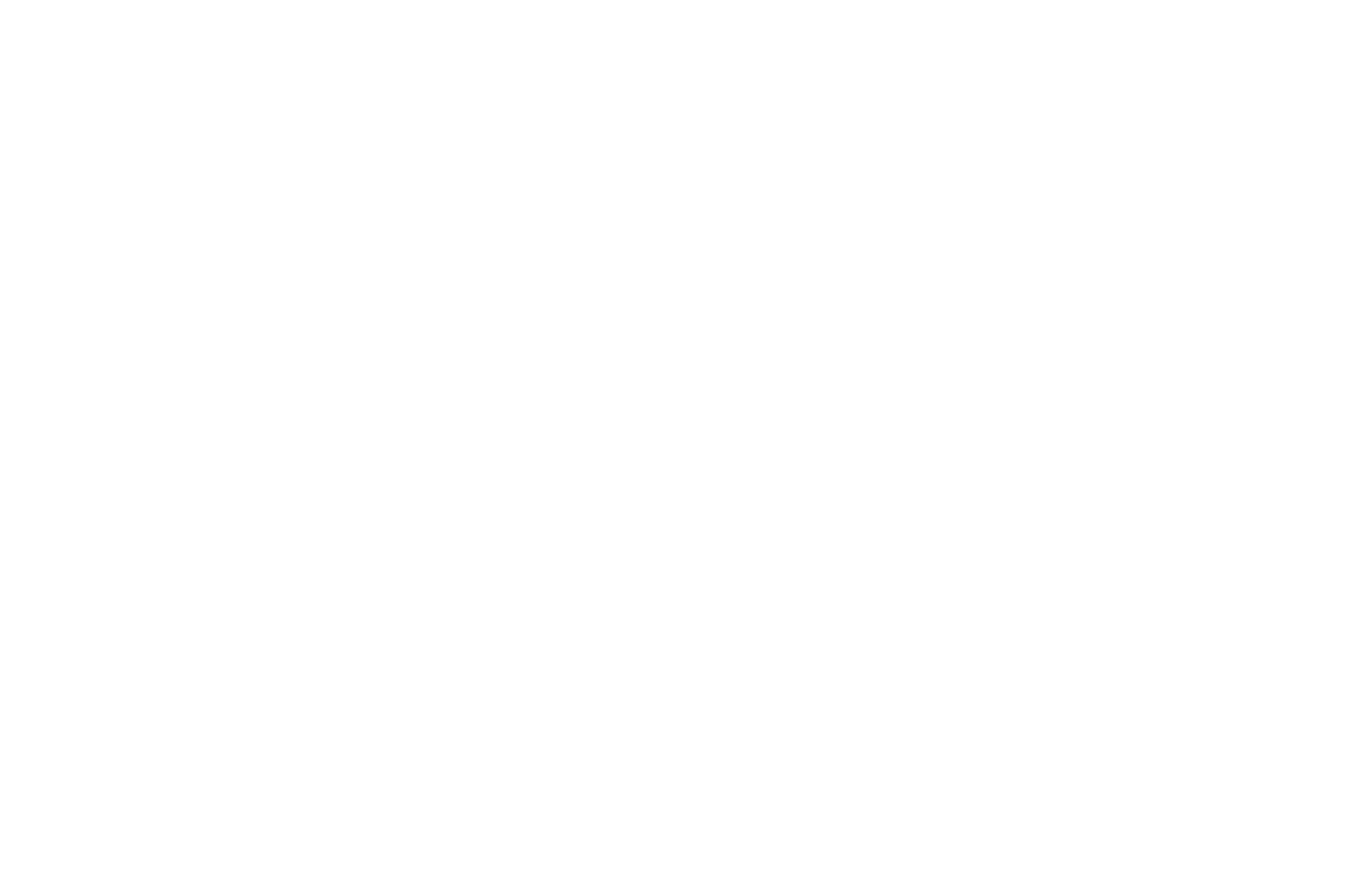
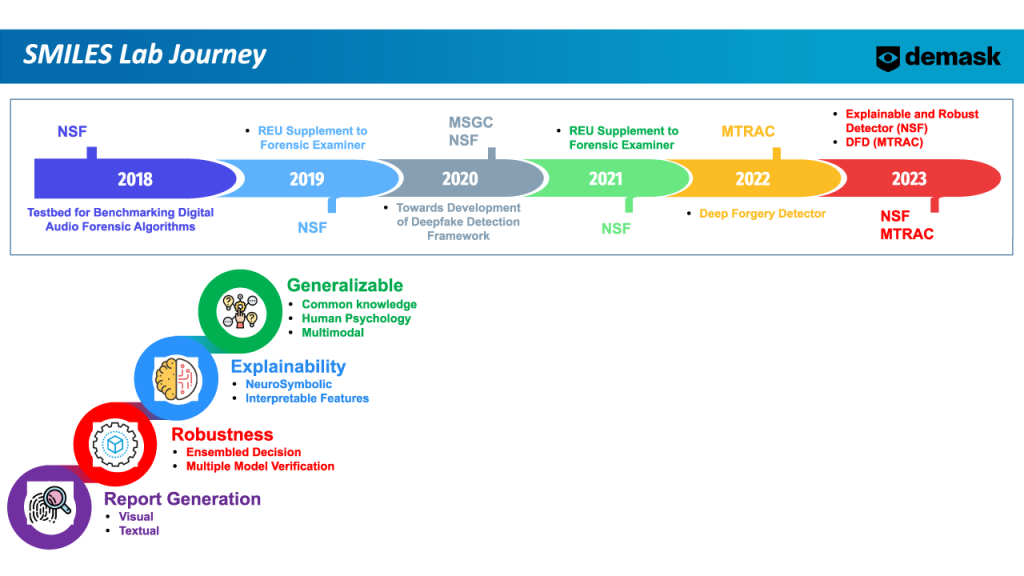
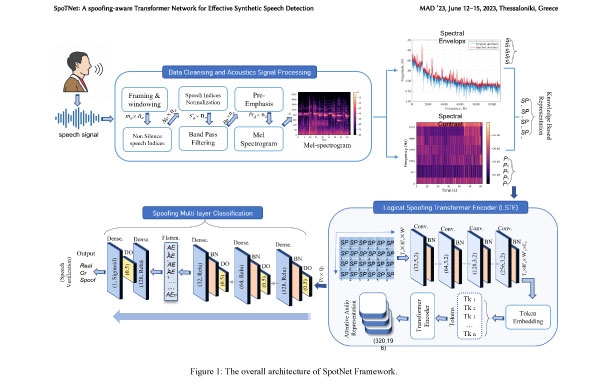
This NSF partnership for innovation (NSF-PFI) and MTRAC-funded project seeks to further improve Deep Forgery Detector (DFD) technology built on NSF lineage award# 1815724: SaTC: CORE: ForensicExaminer: Testbed for Benchmarking Digital Audio Forensic Algorithms and MTRAC project titled “Deep Forgery Detector.” The DFD detects audio-visual forgeries, including various types of Deepfakes, that are used in the manipulation of digital multimedia, but new types are continuously appearing. Improvements to the DFD MVP will help to make it more robust against anti-forensics and also make it more accessible and explainable.
For details, see:
- NSF Award Abstract: ForensicExaminer: Testbed for Benchmarking Digital Audio Forensic Algorithms
- NSF Award Abstract: Deep Forgery Detection Technology
- MEDC Press Release: MTRAC Innovation Hub for Advanced Computing Welcomes Third Cohort of Early-Stage Deep Tech Innovation Projects
NeuroAssist: An Intelligent Secure Decision Support System for the Prediction of Brain Aneurysm Rupture
Funded by the Brain Aneurysm Foundation
Cerebrovascular accident, or stroke, is the leading cause of disability worldwide and the second leading cause of death. Additionally, stroke is the fifth leading cause of death for all Americans and a leading cause of serious long-term disability. Annually, 15 million people worldwide suffer a stroke, and of these, 5 million die and another 5 million are left permanently disabled.
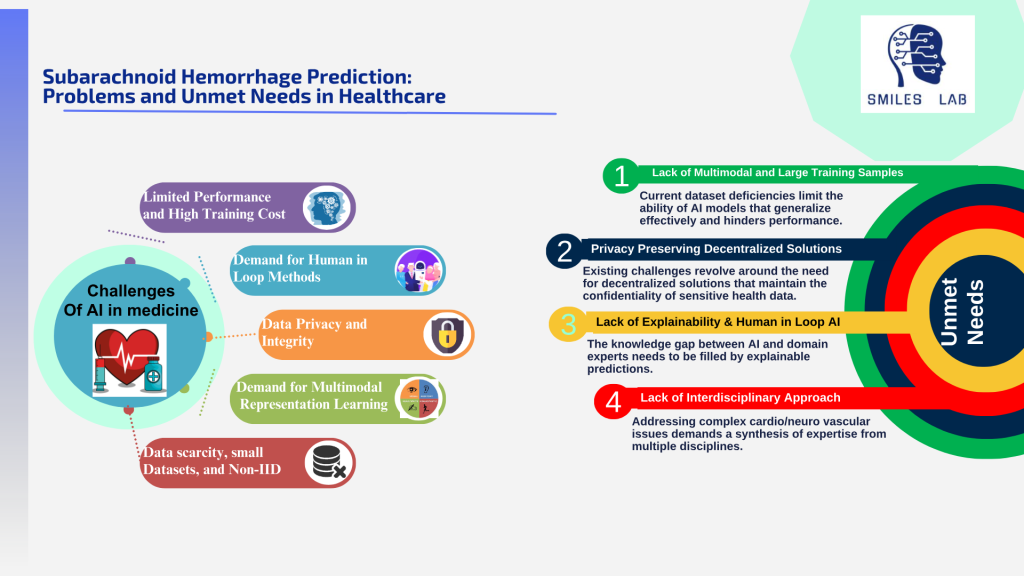
In order to prevent these deaths and disabilities, neurologists and neurosurgeons must be able to diagnose the root causes early and improve their clinical management. They also need to determine an individual’s overall risk across multiple complex considerations, including cerebral aneurysms, arteriovenous malformations (AVM), and Cerebral Occlusive Disease (COD).
Clinical management of diseases causing stroke is very complex. To illustrate the complexity, take the factor of Unruptured Intracranial Aneurysms by itself. Treating them is a complex decision-making process because the risk of rupture is not solely determined by the size of the aneurysm. Location/artery matters a great deal; small aneurysms on certain arteries may rupture, while larger ones on other arteries may not.
Beyond the isolated case of the aneurysms themselves, various degrees of arteriovenous malformations and plaque accumulation inside the carotid arteries can add other risk factors to the overall stroke risk. Our current assessments are not enough to meet this complexity.
Lack of proper data often leads to a decision-making process that could aptly be described as ‘better safe than sorry.’ It is certainly true that surgical intervention is a successful method for eliminating the risk of stroke. However, these surgeries are invasive and may result in severe iatrogenic complications or neurological deficits so treating all aneurysms/AVMs/COD is not always worth that risk.
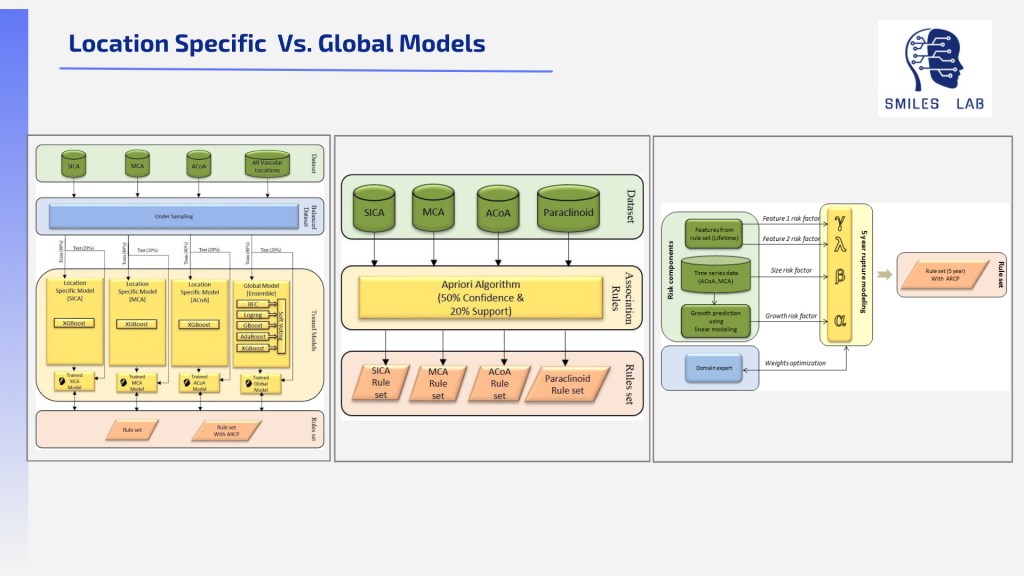
On the other hand, delayed intervention when combining factors increases the risk of a stroke, the consequence can be death or permanent disability. When the overall risk is high, it is imperative to perform the correct treatment right away.
Without a dependable clinical risk/severity score available, neurosurgeons must rely on heuristics compiled from unreliable data and their previous experience.
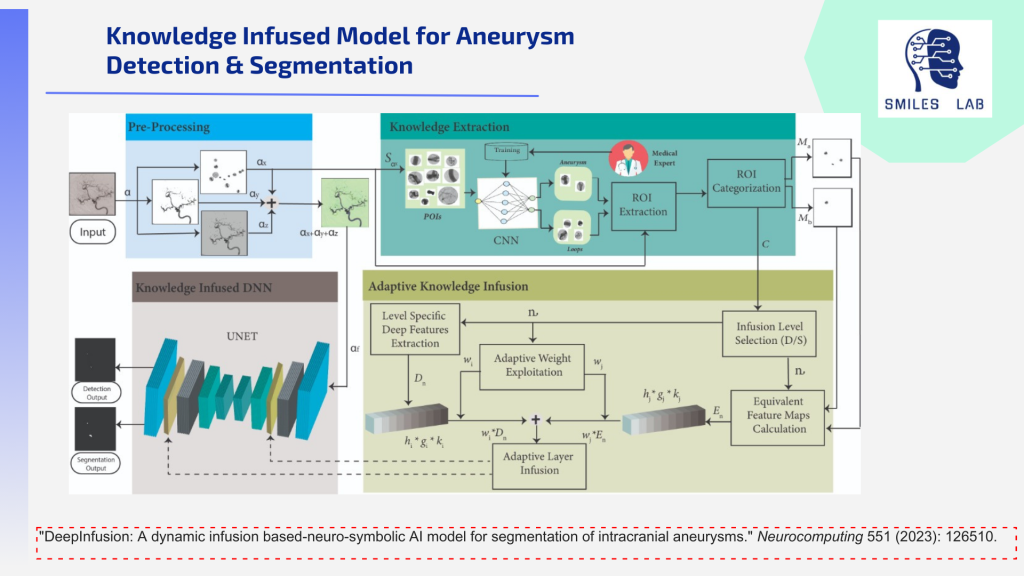
Between those two extremes are many cases where the risk warrants monitoring over time, not action. Doctors struggle with the decision of when to treat and when to watch, and every year thousands of unnecessary procedures are performed because they just aren’t. sure. Quantifying the overall stroke risks based on a group of risk factors in similar patients can help make this crucial decision much easier for neurosurgeons.
This means the tool needs to be a trusted one that clinicians can use to explain the individual situation. The patient and family are imagining the worst outcomes. They are worried about a devastating stroke and the financial burden of treatment. Being able to clearly explain why the best option is to wait and monitor would be a wonderful benefit to those families.
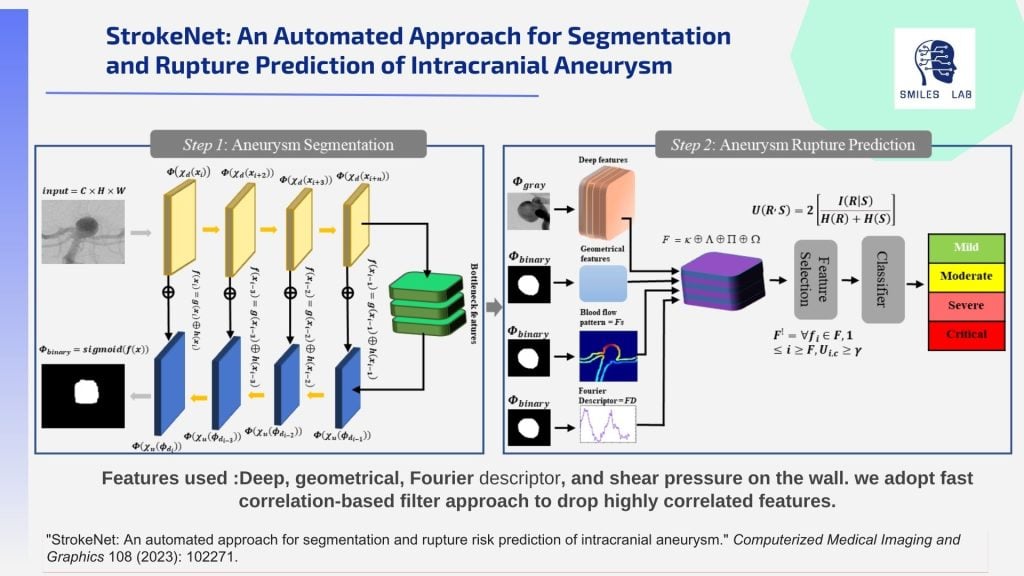
To meet this need, we have developed tools with a decentralized and highly explainable AI-based approach. These tools use a wide array of techniques: Multimodal AI on Digital Subtraction Angiography, Magnetic Resonance Angiography, and Computed Tomography Angiography image modalities along with clinical text, federated learning, RAG-based Neuro-symbolic AI, computational fluid dynamics, and multimodal explainable AI.
Ultimately, this project will deliver tools that will reduce fatalities and long-term disabilities, defray high costs for patients and our healthcare system, and alleviate much psychological stress for patients. It will also help to develop and share more robust data with other researchers to advance our understanding of brain aneurysms going forward.
For details, see: Brain Aneurysm Foundation: Meet Research Grant Recipient: Khalid Malik, PhD
Neuro-symbolic AI-based Web Filtering
Sponsored by Netstar Inc.
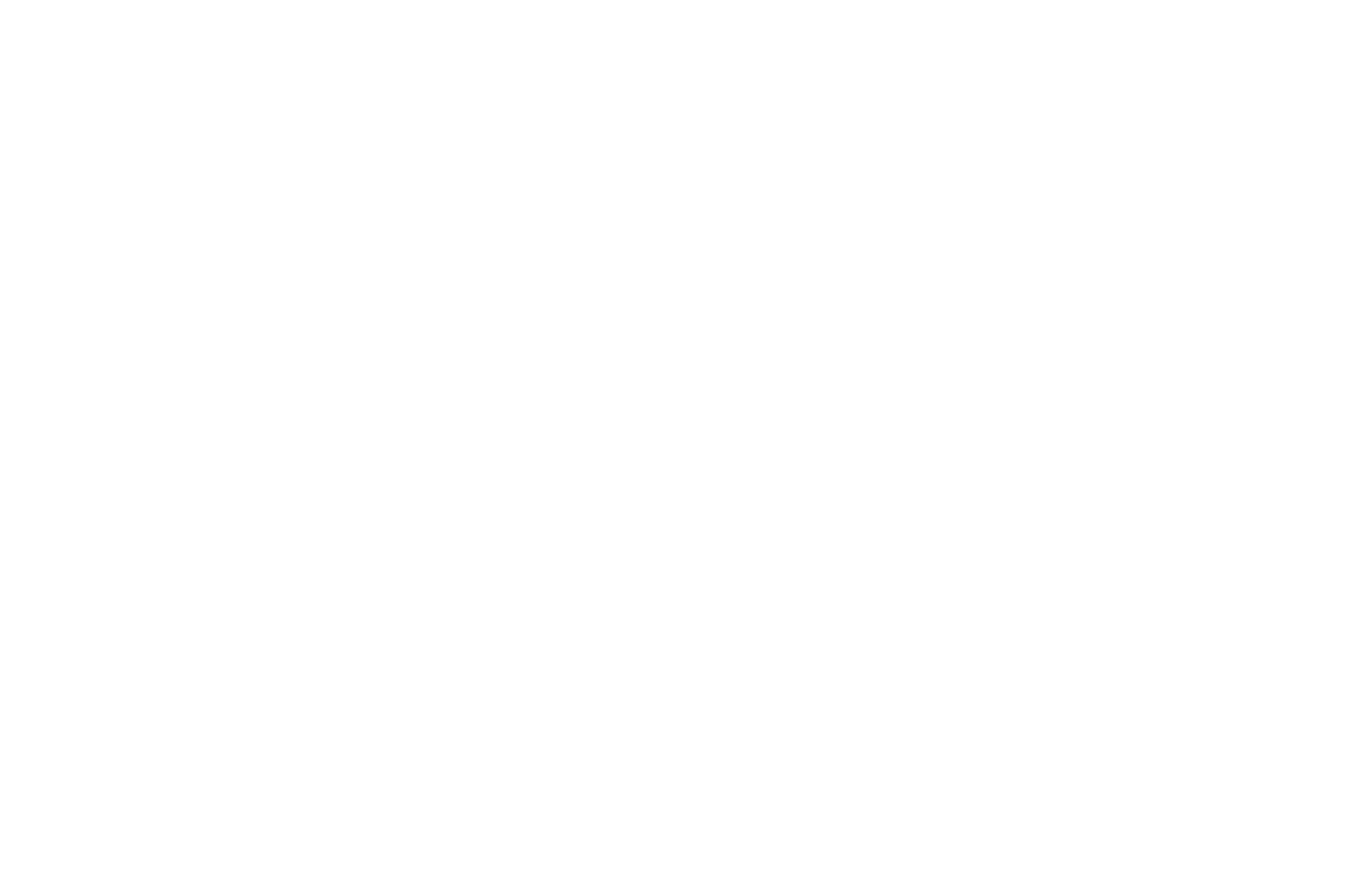
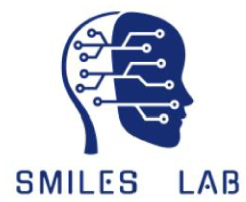

Explainable Multimodal Neurosymbolic
Edge AI Models for Web Filtering
Secure Modeling and Intelligent Learning in Engineering Systems Lab
Web filtering solutions are a vital component of cybersecurity. They block access to malicious websites, prevent malware from infecting our machines, and protect sensitive data from going out of organizations. They offer a secure, efficient, and controlled online experience across various sectors, addressing concerns related to security, productivity, and content appropriateness. The growing trends in Internet usage for data and knowledge-sharing calls for dynamic classification of web contents, particularly at the edge of the Internet.
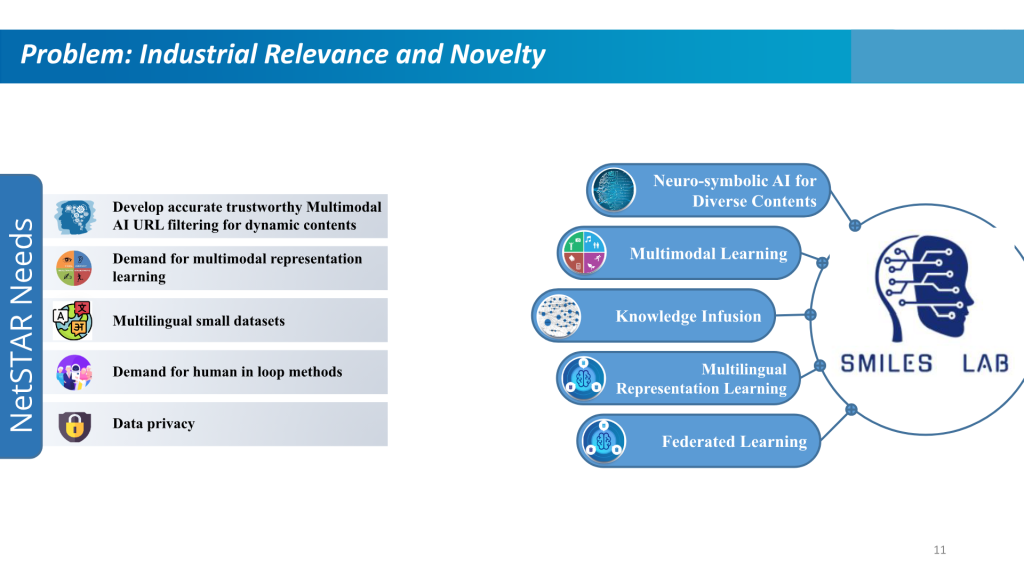
Companies today need these solutions to have multilingual capabilities and protect the data privacy of their employees. To meet these challenges requires a reliable solution that can effectively classify the URLs into correct classes.
To meet these needs, UM-Flint has partnered with leading Japanese URL Filtering company, Netstar Inc., to develop a machine learning-based solution. The team consists of multiple PhD and postdoc students of Secure Modeling and Intelligent Learning in Engineering System (SMILES) Lab and employees of Netstar.
Students involved in this project will learn advanced techniques of Natural Language Processing, multilingual content processing, and development of knowledge graphs. They will gain experience with neurosymbolic and multimodal AI that is explainable and offers reasoning. They will also have opportunities to gain the many soft skills required for collaboration with a global corporation.
Automated Neuro Knowledge Graph Curation
To develop Neurosymbolic AI systems, it’s essential to have knowledge graphs that represent all the entities of the domains and the relationships between them.
The rapid growth of Knowledge Graphs (KG) in recent years has been indicative of a resurgence in knowledge engineering. The use of KGs in the published literature to distill usable information that neuro-symbolic models and expert-based systems could use is one of the most promising approaches to the data consumption problem; and also, it provides more explanations for AI techniques such as machine learning and deep learning
Most companies today recognize that data is their most valuable asset, but it can come in many different forms and formats. Making that data usable for ML and AI tools is challenging. Currently, knowledge graph creation and curation are mostly manual or somewhat semi-automated, and thus it is a labor-intensive process. In many cases, this manual process takes a person with a high level of expertise away from investing that time in the core product or scientific work they could be doing.
The automated curation of knowledge graphs from voluminous unstructured data can extract actionable information that is machine-readable and can potentially help knowledge discovery from Big data. To get actionable information, it’s necessary to identify sources and meanings of and relationships between entities of the given domains.
Furthermore, automatically extracting reliable and consistent knowledge particularly from structured and unstructured sources at scale is a formidable challenge. Very few attempts have been made on the automated construction of health knowledge graphs. The ones that have been tried limited their focus to the creation of triplets by having only one type of relationship.
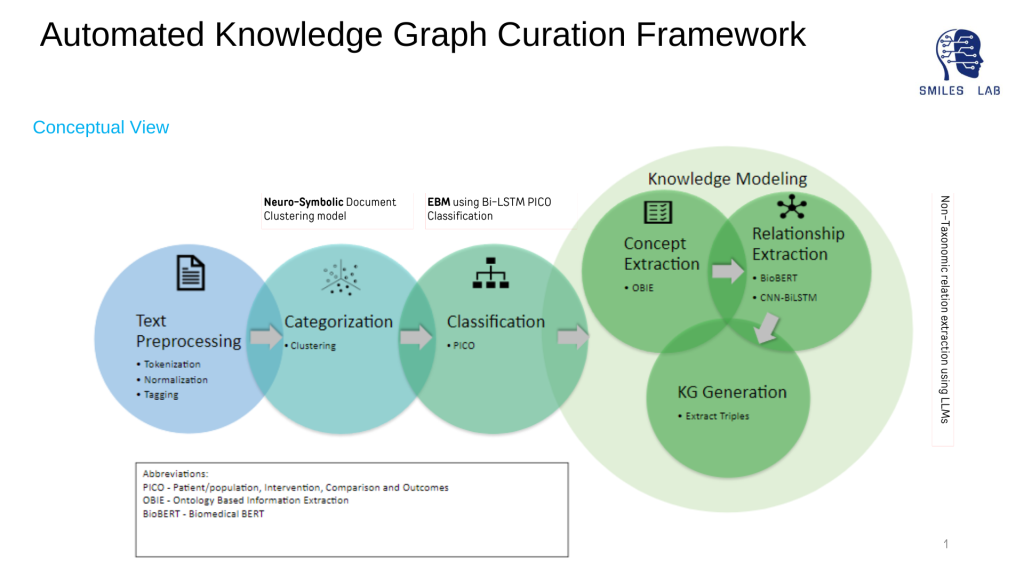
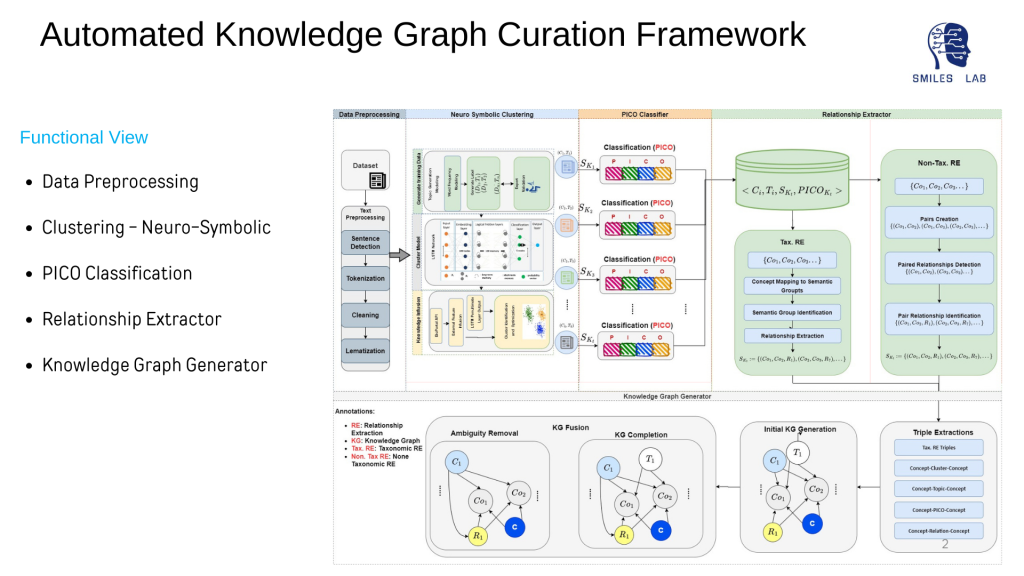
Traditional models do not consider semantic, correlative, and causal relationships among domain concepts in knowledge graphs. None of the existing approaches have focused on building hierarchical relationships among extracted concepts. Additionally, concept extraction using either word embedding, or ontology-based information extraction does not give reliable accuracy, and this also affects the accuracy of relationship extraction. Lastly, efforts have not been made to develop predictive knowledge that should be interpretable to both machines and humans to enable true symbiotic human-machine and machine-machine interactions.
This project attempts to solve the above-mentioned challenges by proposing an automated domain-specific knowledge graph construction by making use of structured and unstructured data. This process is being repeated across multiple industries in the Deepfake Detector MVP, NeuroassistAI, and Netstar AI-based web filtering projects.
Automotive Cybersecurity Education
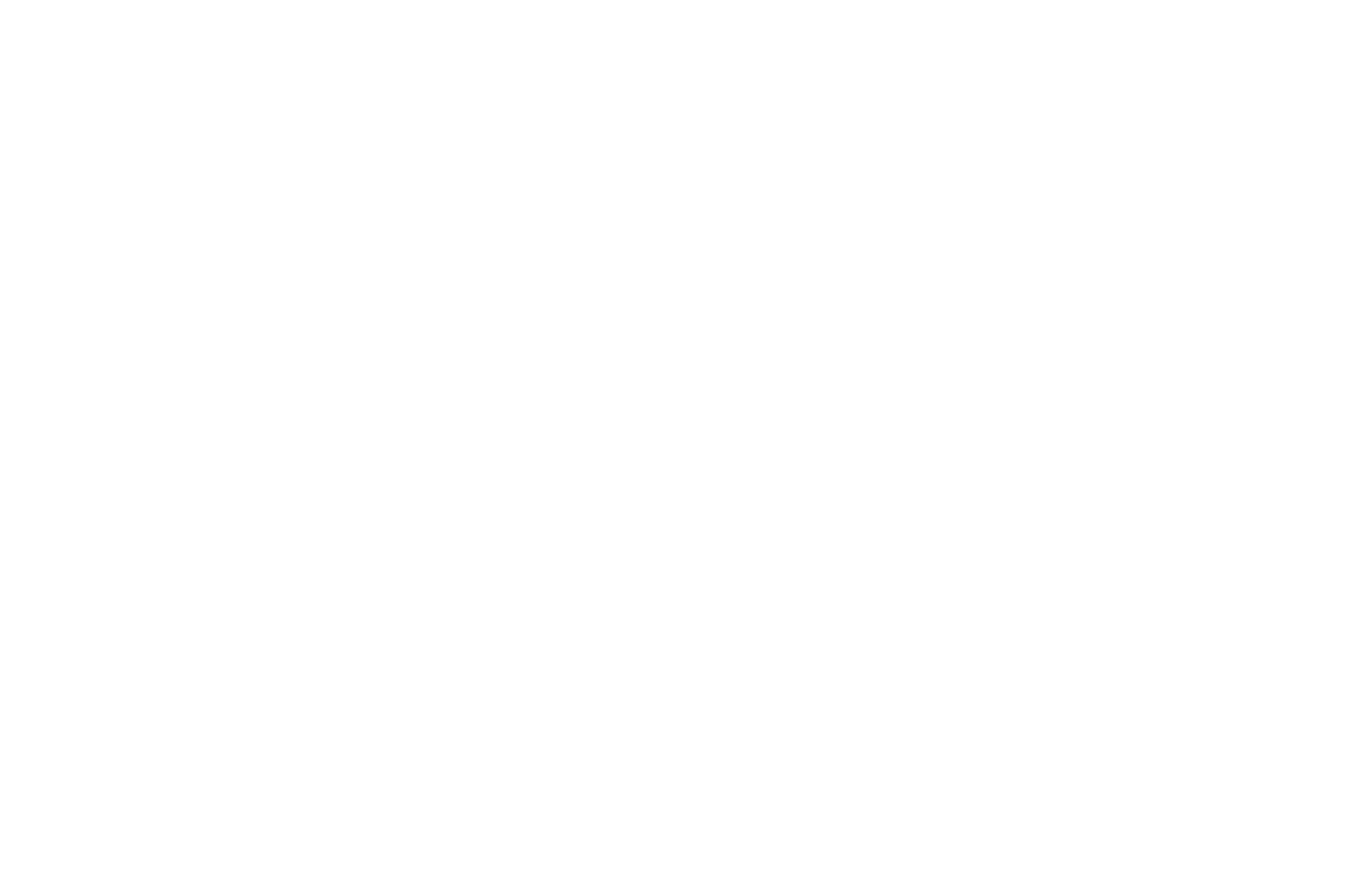
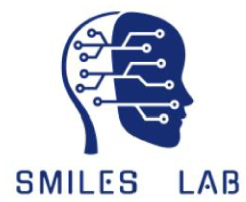

Integrity Verification of Vehicle’s sensor
using Digital Twin and Multimodal AI
Secure Modeling and Intelligent Learning in Engineering Systems Lab
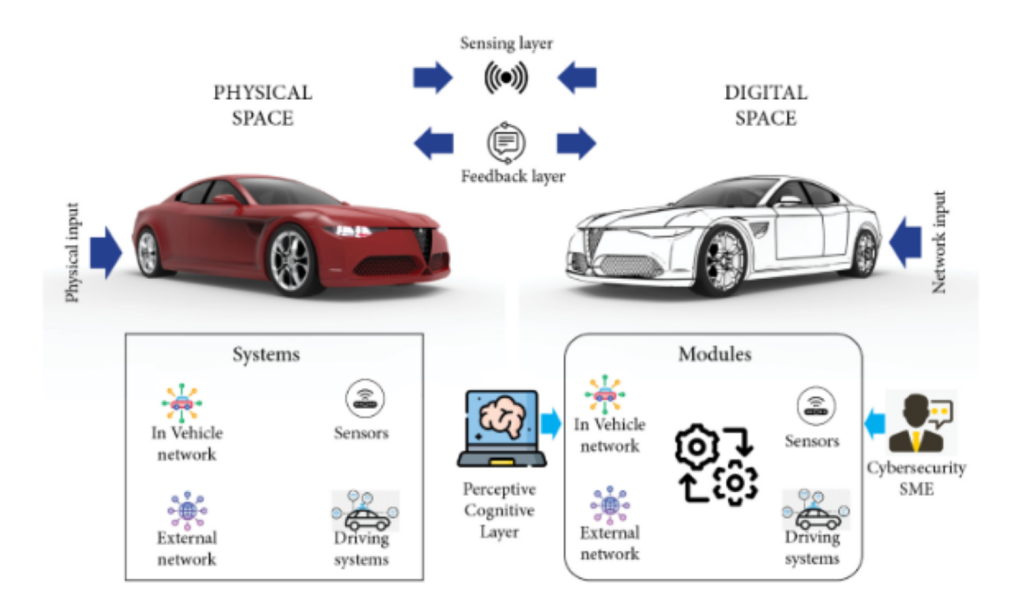
Training and recruiting cybersecurity is one of the most pressing issues in workforce development today. The global Institution of Engineering and Technology has released Automotive Cyber Security, a thought leadership review of risk perspectives for connected vehicles, which explains that our trajectory toward more connected vehicles has greatly increased the need for cybersecurity professionals in the automotive industry.
Filling those roles has a challenge, though: the learning curve. Cybersecurity education as it’s done today can be a little dry and theoretical, making it seem more inaccessible than it actually is, but it doesn’t have to be that way.
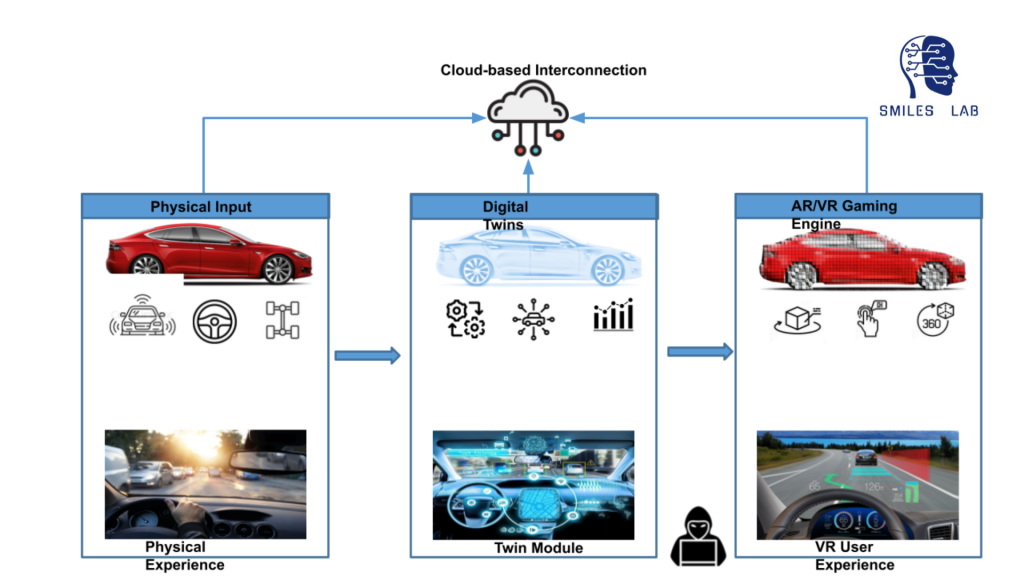
Our research group is developing various tools such as virtual ‘digital twins’ and a visual question-answer system to teach the complexity of interdisciplinary subjects such as cybersecurity in automobiles. This process will enable students to have a VR experience of the complex ways that IoT sensors, the driving systems, and the networks of systems and software in and out of the vehicle interact in a physical vehicle.
In summary, using neuro symbolic logic, AI and the flipped classroom, we’re working to redesign classes from the ground up, starting with a new offering in automotive cybersecurity. The class will feature hands-on exercises on the digital twin of a real car system and will also offer 24/7 assistance with a chatbot based on a large language model like chatGPT.